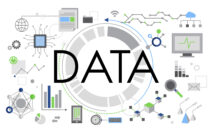
Table of Contents
Discover how to simplify data governance and integration within the insurance sector. Learn key strategies and best practices to streamline your processes and enhance efficiency.
I. Introduction
In today’s data-driven world, the insurance sector is experiencing a fundamental shift. The industry is collecting and processing more data than ever before, from customer information to claims data, and the need for effective data governance and integration strategies is paramount.
In this blog post, we will explore the challenges and opportunities surrounding data governance and integration in the insurance sector and discuss best practices for ensuring data quality, security, and compliance.
Data governance refers to the process of managing data assets within an organization. It involves the overall management of data, including data quality, data access, data security, data privacy, and compliance with regulatory requirements.
II. Importance of Data Governance in the Insurance Sector
1. Data Quality: Insurance relies heavily on accurate and reliable data. Data governance ensures that the data used for underwriting, claims processing, and risk assessment is of high quality and integrity. Inaccurate data can lead to significant financial losses and harm an insurer’s reputation.
2. Regulatory Compliance: The insurance industry is subject to a multitude of regulations, varying by region and type of insurance. Effective data governance helps insurance companies comply with these regulations, such as GDPR, HIPAA, and industry-specific laws. Failure to comply may result in hefty fines and legal repercussions.
3. Risk Mitigation: Insurance companies are exposed to various risks, including fraud, cyber threats, and market fluctuations. Proper data governance reduces these risks by ensuring data security, monitoring for anomalies, and maintaining historical data for risk analysis.
4. Customer Trust: Policyholders entrust insurance companies with sensitive personal information. Effective data governance builds and maintains trust by safeguarding this data from breaches and misuse.
III. Exploring the Regulatory Landscape and its Impact
1. Privacy Regulations: Data privacy regulations, such as DPDP in India, GDPR in Europe and CCPA in California, require insurers to protect policyholder data, ensure data subject rights, and report data breaches promptly.
2. Anti-Fraud Regulations: Insurance regulators demand measures to prevent and detect insurance fraud, making data analysis and fraud detection a critical component of data governance.
3. Data Retention Regulations: Regulations dictate how long insurers must retain data, which may vary depending on the type of insurance and the jurisdiction. Data governance ensures proper data retention practices.
4. Market Conduct Regulations: Insurers must comply with rules governing marketing practices, product development, and consumer interactions, which often involve data usage and analytics.
IV. Top Risks of Poor Data Governance
Data governance is pivotal in the insurance sector for ensuring data accuracy, complying with regulations, mitigating risks, and maintaining customer trust. Poor data governance can result in financial, legal, and reputational consequences, making it imperative for insurers to invest in robust data governance practices.
Poor data governance in the insurance sector can have severe consequences.
1. Financial Loss: Inaccurate data, whether in underwriting, claims processing, or pricing, can lead to financial losses due to overpayment, underpayment, or mispricing of policies.
2. Regulatory Non-Compliance: Failing to adhere to data-related regulations can result in substantial fines and damage the reputation of an insurer.
3. Security Breaches: Inadequate data security measures can lead to data breaches, exposing policyholder information to unauthorized access and increasing the risk of identity theft and fraud.
4. Inefficient Operations: Without proper data governance, insurance processes become inefficient and time-consuming, leading to increased operational costs.
5. Loss of Trust: Poor data governance practices erode policyholder trust, as they question the security and privacy of their data.
V. Top data sources in insurance sector
The insurance industry relies on a wide variety of data sources, ranging from traditional legacy systems to emerging technologies like IoT devices:
1. Legacy Systems: Many insurance companies still use legacy systems that were not designed for modern data integration. These systems often store data in formats that are incompatible with newer technologies.
2. IoT Devices: The proliferation of IoT devices, such as telematics in vehicles and smart home sensors, has led to a massive influx of real-time data. Insurers can use this data for risk assessment and pricing.
3. Third-Party Data: Insurers often utilize data from external sources, like credit bureaus or weather data providers, to make more accurate underwriting and claims decisions.
4. Customer Portals: Policyholders frequently interact with insurance companies through web portals and mobile apps. These interactions generate data that can be valuable for improving customer service and understanding customer behavior.
5. Agents and Brokers: Data generated by agents and brokers in the form of applications, contracts, and communications is crucial for policy issuance and management.
VI. Challenges in Integrating Data from Sources
1. APIs (Application Programming Interfaces): APIs allow different systems to communicate and share data. Insurance companies can use APIs to connect legacy systems with modern platforms and external data sources.
2. ETL Processes (Extract, Transform, Load): ETL processes involve extracting data from source systems, transforming it into a compatible format, and loading it into a data warehouse or data lake. ETL tools can help automate these processes.
3. Data Lakes: Data lakes are storage repositories that can hold vast amounts of structured and unstructured data. They are flexible and allow for the storage of diverse data types, making it easier to access and analyze.
4. Data Integration Platforms: Specialized data integration platforms are available that cater to the insurance industry. These platforms offer pre-built connectors for common insurance systems and help streamline data integration.
5. Data Governance: Effective data governance practices help ensure data is accurate, consistent, and well-documented. This is essential for integration success.
6. Real-Time Processing: For real-time data from IoT devices, insurers can invest in technologies that enable real-time processing, analysis, and decision-making.
In conclusion, data integration in the insurance sector is essential for leveraging the wealth of information available from various sources. Challenges like data silos and diverse data formats can be overcome through APIs, ETL processes, data lakes, and specialized data integration platforms. Successful data integration is key to making informed underwriting decisions, enhancing customer service, and optimizing business processes.
VII. The Role of Data Analytics and AI in the Insurance Sector
Data analytics and artificial intelligence (AI) play a crucial role in the insurance sector, helping insurers extract actionable insights and make data-driven decisions:
1. Risk Assessment: AI and data analytics can assess risk more accurately by analyzing a broad range of data sources, including IoT data and historical claims data. This allows insurers to refine underwriting and pricing models.
2. Claims Processing: Automated claims processing using AI can expedite the settlement of claims, reducing operational costs and enhancing customer satisfaction.
3. Customer Insights: Analytics tools can provide insurers with a deeper understanding of customer behavior and preferences, enabling personalized marketing and product recommendations.
4. Predictive Analytics: Insurers can use predictive models to anticipate future trends, claims, and customer needs, allowing them to proactively respond and stay ahead of the competition.
5. Fraud Detection: AI algorithms can detect anomalies and patterns indicative of fraud, helping insurers prevent fraudulent claims and minimize financial losses.
VIII. Examples of Insurers Benefiting from Data-Driven Insights
1. Progressive Insurance: Progressive uses telematics data from customer vehicles to offer usage-based insurance. By analyzing this data, they can offer personalized pricing based on driving habits, attracting safer drivers and gaining a competitive edge.
2. Lemonade: Lemonade, a digital insurance startup, uses AI and data analysis to streamline the claims process. They boast rapid claims resolution times, setting them apart in the industry and gaining a competitive advantage in customer service.
3. AXA: AXA uses data analytics to understand customer behavior and preferences. This helps them tailor insurance products and services to individual needs, providing a competitive edge in customer engagement and satisfaction.
4. Geico: Geico employs data-driven advertising and personalized marketing strategies based on customer data analysis. This approach has helped them stand out in a crowded market and gain a competitive edge in customer acquisition.
In summary, insurance companies that effectively implement data governance, integration, data analytics, and AI can gain a competitive edge by making more informed decisions, improving customer service, increasing efficiency, and proactively managing risk. Several insurers have already demonstrated the benefits of data-driven insights in the industry, setting examples for others to follow.
IX. Best Practices for Data Governance and Integration
1. Clearly Define Data Ownership: Assign responsibility for data quality and security to specific individuals or teams within the organization. Ensure that they have the authority to enforce data governance policies.
2. Develop Data Governance Policies: Create comprehensive data governance policies and procedures that cover data quality, security, privacy, and compliance. Ensure that all employees are aware of and trained on these policies.
3. Data Classification: Classify data based on sensitivity and importance. This helps in prioritizing data governance efforts and ensuring that sensitive data receives the highest level of protection.
4. Data Quality Standards: Establish data quality standards and implement data validation processes to ensure data accuracy and consistency. It’s important to monitor data quality on a regular basis and address issues promptly.
5. Data Security Measures: Employ strong data security measures, including encryption, access controls, and regular security audits, to protect sensitive data from breaches.
6. Assess Integration Needs: Begin by understanding your organization’s unique integration requirements. Consider the types of data sources, data volumes, and the need for real-time data access.
7. Evaluate Integration Platforms: Research and evaluate integration platforms that align with your organization’s requirements. Look for tools that offer scalability, flexibility, and compatibility with your existing systems.
8. Consider APIs: Application Programming Interfaces (APIs) provide a flexible way to connect systems and data sources. Evaluate APIs for their ease of use, performance, and security.
9. ETL and Data Lakes: If dealing with large volumes of data, consider Extract, Transform, Load (ETL) processes and data lakes. ETL tools help prepare and move data, while data lakes provide storage and analytics capabilities.
10. Cloud Integration: Assess the benefits of cloud-based integration platforms, which can provide scalability, cost-effectiveness, and the ability to leverage cloud-native services.
11. Continuous Data Quality Monitoring: Implement continuous data quality checks to ensure data remains accurate and consistent. Automate data quality monitoring whenever possible.
12. Regular Audits: Conduct periodic audits of data governance and integration processes to identify vulnerabilities, assess compliance, and make necessary adjustments.
13. Training and Awareness: Keep employees informed about data governance best practices and the importance of data integration. Regular training sessions can help maintain a data-aware culture.
14. Data Privacy Compliance: Stay updated on evolving data privacy regulations and ensure that data governance practices align with legal requirements.
15. Feedback Loop: Establish a feedback loop for employees to report data issues or integration challenges. Use this feedback to continually improve data governance and integration processes.
X. Predicting Future Trends in Data Governance and Integration
1. Increased Emphasis on Data Ethics: With growing concerns around data privacy, insurers will need to invest more in ethical data practices. This includes transparent data usage, consent management, and responsible AI.
2. AI-Driven Automation: AI and machine learning will play a larger role in automating data integration, analysis, and decision-making processes, improving efficiency and accuracy.
3. Blockchain for Trust: Blockchain technology will be used to create secure, immutable records of insurance transactions, enhancing trust between insurers and policyholders.
4. Real-Time Data Processing: As the Internet of Things (IoT) expands, insurers will need to handle real-time data from connected devices, making real-time data processing and analytics more critical.
5. Data-As-A-Service (DaaS): The concept of DaaS will gain traction in the insurance industry, enabling insurers to access third-party data sources in real-time, enriching their risk assessment and underwriting processes.
XI. Emerging Technologies and Their Potential Impact
1. 5G Networks: The rollout of 5G networks will enable faster data transmission, making it easier to collect and process data in real-time from IoT devices.
2. Edge Computing: Edge computing will bring data processing closer to the data source, reducing latency and enabling quicker decision-making in insurance applications.
3. Explainable AI: As AI becomes more prevalent, insurers will rely on explainable AI models to understand and justify automated decisions, ensuring compliance and transparency.
4. Quantum Computing: In the long term, quantum computing has the potential to revolutionize data processing, significantly enhancing complex risk assessments and simulations.
5. Digital Twins: Insurers can create digital replicas of real-world assets (e.g., buildings, vehicles) to assess risk and monitor changes in real-time, using IoT data.
XII. Encouraging Insurers to Adapt and Innovate in the Data-Driven Landscape
Insurers should embrace the following strategies to adapt and innovate in the data-driven landscape:
1. Cultivate a Data-Centric Culture: Foster a culture of data awareness and innovation within the organization. Encourage employees to see data as a strategic asset.
2. Invest in Talent: Hire and train data scientists, data engineers, and analytics experts to harness the full potential of data.
3. Collaborate with Insurtechs: Partner with insurtech startups to leverage their expertise in data analytics, AI, and emerging technologies.
4. Stay Informed: Stay informed about evolving regulations, especially regarding data privacy, and adapt data governance practices accordingly.
5. Experiment and Iterate: Don’t be afraid to experiment with new data sources and technologies. Experimentation can lead to valuable insights and new product offerings.
6. Customer-Centricity: Use data to better understand customer needs and preferences, offering tailored products and services that set you apart in a competitive market.
XIII. Conclusion
In conclusion, the future of data in insurance will be marked by ethical data practices, advanced technologies, and real-time data processing. To thrive in this landscape, insurers must adapt, innovate, and make data-driven decisions at every level of their operations. By doing so, they can provide better services, gain a competitive edge, and meet the evolving demands of policyholders and regulators.
Incorporating these best practices into your data governance and integration strategies will help your organization maintain data quality, security, and compliance while achieving greater efficiency and competitive advantage. Remember that data governance and integration are ongoing processes that require attention and adaptation as technology and business needs evolve.
Read more on https://cybertechworld.co.in for insightful cybersecurity related content.
Thank you for your sharing. I am worried that I lack creative ideas. It is your article that makes me full of hope. Thank you. But, I have a question, can you help me?