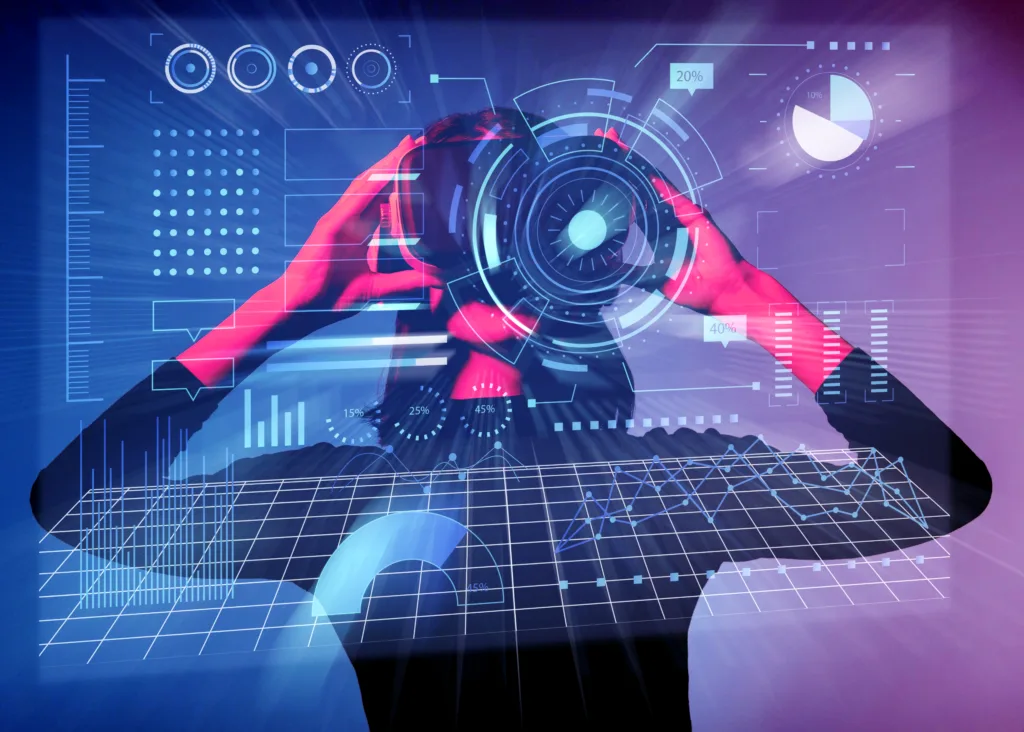
Table of Contents
In the ever-evolving landscape of cybersecurity, where threats are becoming increasingly sophisticated, traditional defense mechanisms often fall short. As the digital realm expands, so does the complexity of potential risks.
In this blog post, we embark on a deep dive into the world of cybersecurity to unravel how deep learning, a subset of artificial intelligence, is revolutionizing our approach to safeguarding sensitive information and digital assets.
The Current State of Cybersecurity
Before we delve into the transformative power of deep learning, let’s assess the current state of cybersecurity. Traditional methods, relying heavily on rule-based systems and signature-based detection, are struggling to keep pace with the dynamic nature of cyber threats.
Hackers are employing advanced tactics, adapting to circumvent conventional defenses, leaving organizations vulnerable to attacks that can compromise data integrity, confidentiality, and availability.
Enter Deep Learning In Cybersecurity
Deep learning represents a paradigm shift in cybersecurity. At its core, deep learning is a subset of machine learning that employs neural networks with multiple layers (deep neural networks) to analyze and make sense of vast amounts of data.
Its ability to identify patterns, learn from experience, and make predictions renders it a powerful tool in the fight against cyber threats.
1. Anomaly Detection
Traditional cybersecurity systems often rely on known signatures of malware or predefined rules to detect threats. Deep learning, on the other hand, excels in anomaly detection. By learning the baseline behavior of a system, it can identify deviations that may indicate a potential threat. This proactive approach enables the detection of previously unknown and emerging threats, providing a crucial edge in cybersecurity defense.
2. Zero-Day Threat Detection
Zero-day threats, exploiting vulnerabilities unknown to the software vendor, pose a significant challenge to cybersecurity. Deep learning’s ability to recognize patterns beyond predefined rules makes it well-suited for identifying and mitigating zero-day threats. Its adaptive nature allows it to learn from new data and adjust its models, providing real-time defense against evolving attack vectors.
3. Advanced Phishing Detection
Phishing attacks continue to be a prevalent and effective method for cybercriminals to gain unauthorized access. Deep learning algorithms can analyze patterns in email content, sender behavior, and other factors to identify phishing attempts. By learning from historical data, deep learning models can discern subtle cues that may escape traditional detection methods, enhancing the resilience against phishing attacks.
4. Behavioral Analysis
Understanding normal user behavior is crucial in identifying abnormal activities that may indicate a security threat. Deep learning excels in behavioral analysis by learning patterns associated with legitimate user activities. This approach allows for the detection of insider threats, where compromised or malicious users may exhibit behavior deviating from their usual patterns.
5. Adaptive Defense Mechanisms
One of the remarkable aspects of deep learning is its ability to adapt and evolve. As cyber threats continually evolve, having a defense mechanism that can learn and adjust is invaluable. Deep learning models can be trained on new data, allowing them to adapt to emerging threats and enhance their predictive capabilities over time.
Real-World Applications of Deep Learning in Cybersecurity
Now that we understand the theoretical underpinnings, let’s explore how deep learning is making a tangible impact in real-world cybersecurity scenarios.
1. Network Security
Deep learning is increasingly being used to fortify network security. By analyzing network traffic patterns, it can detect unusual activities that may indicate a cyber attack. This proactive approach enables the identification of potential threats, such as Distributed Denial of Service (DDoS) attacks or suspicious data exfiltration, in real-time.
2. Endpoint Protection
Endpoints, including individual devices and servers, are common targets for cyber threats. Deep learning solutions can be integrated into endpoint protection systems to provide advanced threat detection. Whether it’s identifying malicious files, detecting unusual user behavior, or preventing the execution of malicious code, deep learning enhances the security posture of endpoints.
3. Cloud Security
As organizations increasingly migrate to cloud environments, securing cloud infrastructure becomes paramount. Deep learning models can analyze large volumes of data generated in cloud environments to detect unauthorized access, data breaches, and other security incidents. This level of visibility is crucial in maintaining the integrity and confidentiality of data stored in the cloud.
4. Application Security
Deep learning plays a crucial role in securing applications by identifying vulnerabilities, detecting anomalies in application behavior, and mitigating potential threats. It can analyze code patterns, user interactions, and application data to uncover security risks, providing a proactive defense against application-layer attacks.
Challenges and Considerations
While the potential of deep learning in cybersecurity is immense, it’s essential to acknowledge the challenges and considerations associated with its implementation.
1. Data Privacy and Ethical Considerations
Deep learning relies heavily on vast amounts of data for training and analysis. This raises concerns about data privacy, especially when dealing with sensitive information. Striking a balance between effective cybersecurity and respecting user privacy is an ongoing challenge that requires careful consideration.
2. Resource Intensiveness
Training deep learning models can be resource-intensive, requiring significant computational power and storage. Organizations need to invest in robust infrastructure to support the implementation of deep learning in cybersecurity effectively.
3. Explainability and Interpretability
The “black box” nature of deep learning models poses challenges in understanding how they arrive at specific conclusions. In cybersecurity, where transparency and accountability are crucial, efforts to enhance the explainability and interpretability of deep learning models are ongoing.
Looking to the Future
As we navigate the complex terrain of cybersecurity, the role of deep learning will continue to evolve. The future holds the promise of even more sophisticated models, improved interpretability, and increased integration into holistic cybersecurity frameworks.
Collaboration between cybersecurity experts, data scientists, and ethical considerations will be key in unlocking the full potential of deep learning as a defender against cyber threats.
Conclusion: Riding the Waves of Innovation
In conclusion, the deep dive into understanding how deep learning enhances cybersecurity reveals a transformative force that is reshaping our approach to digital defense. From anomaly detection to adaptive defense mechanisms, the applications of deep learning are diverse and promising.
As we ride the waves of technological innovation, the synergy between human intelligence and artificial intelligence becomes the cornerstone of effective cybersecurity strategies.
By embracing the capabilities of deep learning, organizations can stay ahead of the ever-evolving cyber threat landscape, fortifying their digital fortresses and ensuring the security and resilience of their valuable assets.
The deep dive into deep learning is not just an exploration of technology; it’s a journey toward a safer and more secure digital future.
Read more on https://cybertechworld.co.in for insightful cybersecurity related content.